With energy codes updating across America, many developers are rightly worried about the rising costs associated with compliance. The ultimate goal of these codes is to reach net-zero-energy buildings by 2030. Simply carrying on in business as usual fashion is a recipe for dramatic increases in cost per square foot. The AEC industry in general relies heavily on the belief that what worked on the last project will work for this one. Most architects and engineers comply with the new energy codes by specifying the most expensive systems, wall types, windows and control options. However, new processes and advanced algorithms are giving owners the ability to optimize for first costs to make better decisions on energy.
Energy modeling is rapidly becoming a key tool to make decisions about the appearance and cost of a building. Many owners are told that their design and engineering teams are well integrated and using the latest technology. Unfortunately, many architects and mechanical engineers still have the “throw it over the wall” mentality when it comes to key decisions about energy. Often a consulting mechanical engineer is not providing the information in a timely manner to the architect. Worse yet, the mechanical engineer may wait until the end of design to test any energy model, leading to the inevitable costly changes to the drawings.
Fortunately, making massing and technology decisions is much easier now, thanks to parametric building models and optimizers that provide rapid feedback. It is vital to make sure that the architect you select uses parametric workflows that allow them to test design decisions. Often, architects will rely on how they have always done it. Changing energy codes require a new way of working.
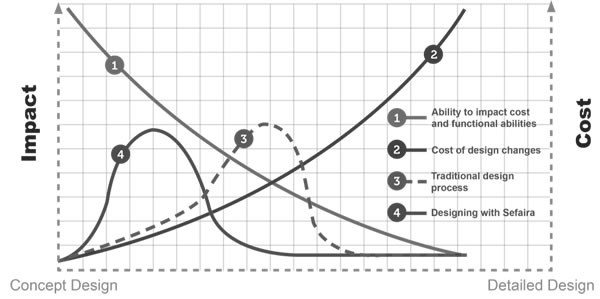
That new way of working is to adopt a big data approach with all the options on the table, using rigorous metrics to ensure that the right decisions are being made. Design objectives such as program, construction cost, environmental performance and aesthetics are key factors in an architectural design. Conceptual design decisions — about a building’s orientation, massing, materials, components and systems — largely determine lifecycle costs of a building. However, with multiple objectives and constraints, the number of decisions quickly spirals out of control. In many cases, limited time and budget limits the set of design options that can be tested during conceptual design. This leads to design solutions with poor initial and lifecycle performance. Thus, owners need architects and engineers who use the power of computation and big data to find low cost solutions that still comply with the code. It will not be possible to build any building without simulation within the next five years.
Interestingly, a parametric approach makes the most financial sense at the beginning of the project when it is easy to make changes. As the design progresses, the cost of changes increases exponentially while the impact of those changes on the cost exponentially decreases. To achieve high-performance design, teams are finding it important to pursue rigorous integration at the early design stage. Owners need to build relationships with architects and engineers who understand the code changes and utilize analytics to make decisions that affect the bottom line.
The first step of the overall process utilizes parametric modeling to represent the parts of the building with editable attributes, and interconnected relationships. Variations of these inputs generate different solutions of the model. Below are four inputs that your architect should vary when seeking a high-performance building.
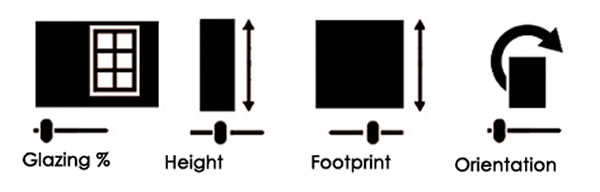
Once these overall variables of glazing percentage, height, footprint and orientation are known, the finer-grained details can be varied further to achieve a high-performance building. As the level of complexity increases, the number of variables considered for analysis should also increase.
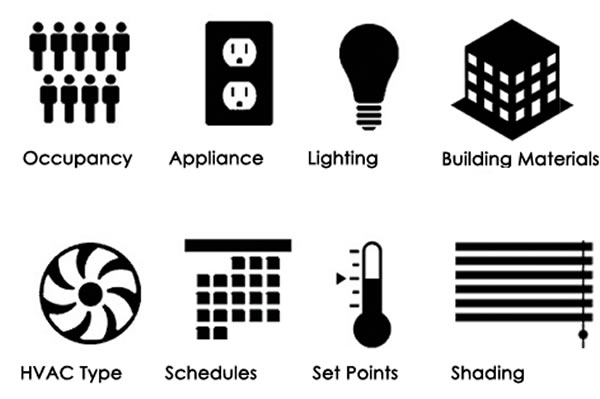
Once the design team has moved forward from the initial massing stage, after considering various alternatives and selecting a smaller list of options, they proceed to the next stage of the design where they make material and technology choices. The manufacturers of building materials, systems and technologies continue to create larger palettes of products with varying performance and cost. This variety allows for a vast array of alternatives available for buildings, resulting in very large numbers of technology combinations.
For example, given 16 technology types, each with three possible options for performance and cost choice, would yield to 4.3 million unique combinations. When a contractor and architect make choices, they are unable to perceive all of the choices and their impacts collectively. This leads to making inefficient choices in terms of either energy or cost or both. This is where, in our Big Data world, cost-vs.-energy optimization can prove useful to get the highest bang for the buck. Below are two case studies showcasing how the use of parametric workflows and cost-vs.-energy optimization yielded higher value to the client in terms of a better and efficient building for a lower cost.
Mass Optimization
A practical application of the process currently under discussion is a new office building near Columbia, S.C. This project is led by a progressive design principal and typifies a type of practice where small agile teams work in an iterative fashion to realize the collective design goal.
The project began with discussions with the client team about their goals and current needs as an organization. This tied directly into choices about concept, site and massing. Incorporating energy early into this process, the figure below demonstrates how the design team iterated over multiple massing ideas looking for the driving factors in the design. Acting as energy analyst on the team, we provided feedback regarding the impact of various building footprints, orientation and glazing percentages (option 1-8) that the design team generated.
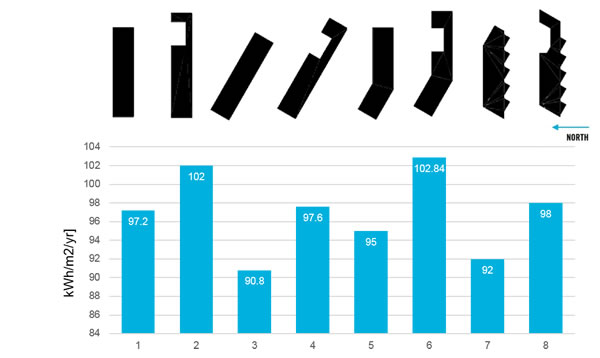
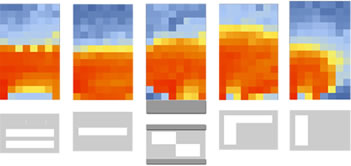
Energy impacts of design decisions are often counterintuitive: The design team found they could enhance the design by rotating the building by 17 degrees off the true north/south orientation to reduce the energy demand by 7 percent. Moreover, the team was able to test multiple glazing percentages by orientation in a single day, in eight working hours.
This energy and daylight feedback proved vital to defining and tracking the client’s energy goals for the project. As the design continued to progress, the energy model kept pace, allowing the tracking of performance across design decisions. The parametric energy analysis process and project team supported the fluid nature of the process by providing feedback on designs with fluctuating levels of detail.
Cost. vs. Energy Optimization
This method also utilizes the fast energy engine for finding the optimal mix of technologies. To illustrate the use of this method, we collaborated with a senior project designer and his team to analyze a 156,000-sq.-ft. (14,492-sq.-m.), eight-story office building in Charleston, S.C., as a case study. As communicated by the design team, this project near the river had employed the rule of thumb approach to come up with a design. All selections were highly typical of office building construction in the Southeast to maximize the functionality of the design.
The building is a typical cast-in-place concrete structure with a continuous glass facade on all sides. As designed, the office building performed just slightly better than the ASHRAE 90.1 2013 baseline. Running the material and technology optimization process on this building yielded an additional cost of $883,065 for a 60-percent energy performance improvement compared to that baseline. Since the overall estimate for the building was $27 million, a 60-percent (4.03 Kwh/sq. ft ) reduction in energy was achieved for a 3.3-percent increase in the estimated cost. With the current price of electricity approximated at 9 cents/kwh, this meant a payback time of 10.4 years. The estimated payback time is likely to decrease with the rise in electricity costs in the upcoming years, demonstrating the achievable impact of this process from an energy and business perspective.
Emerging tools are enabling integrated modules and scripting environments that are narrowing the gaps among architects, engineers and computer programmers. The rapid feedback method can use optimization to create a design space to select materials and technology options while balancing cost vs. percent of energy savings. Building upon this first step, the mass optimization process enabled the generation and analysis of orders of magnitude more design alternatives, thus allowing the exploration of designs that were substantially more energy efficient than those typically evaluated using current methods, at negligible additional process cost.
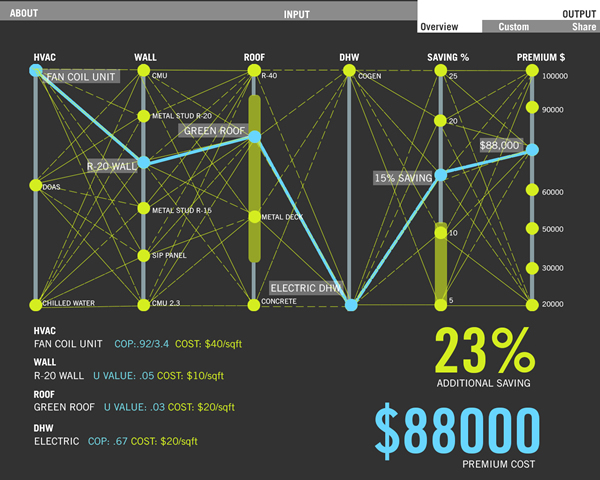
The material and technology optimization allowed the design team to analyze 186 more material and technology combinations than the typical three or four, for a 60-percent energy savings and eight years payback time at minor additional process cost. This can yield highly accurate and useful results with an accurate and diverse cost estimate. Since contractors often offer only one material and technology pricing option, the material and cost optimization is approximated using industry standard cost estimating tools such as RS Means. Future suggestions include proposing the creation of an integrated contractor and mechanical engineering team to allow for more accurate inputs to increase the accuracy of the optimization process and reduce any duplication of work.
Owners and developers must be savy about what kind of architects they hire in the coming years. Many firms talk a good game but are struggling with workflows that are unresponsive to the big data environment in the AEC industry. The owner’s architect should be able to provide designs that feature not only fancy graphics but hard data for appropriate decision making.
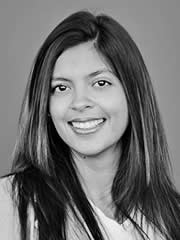
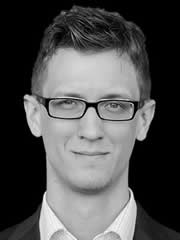
Pattern r+d, founded in 2014, enables the design of sustainable and healthy building environments and communities by developing and applying innovative tools, processes and education. Under the direction of Sandeep Ahuja and Patrick Chopson, Pattern continually reimagines the role of the energy and daylighting consultant. Pattern delivers highly integrated design solutions that go beyond shallow notions of sustainability to true parametric and performance driven design.